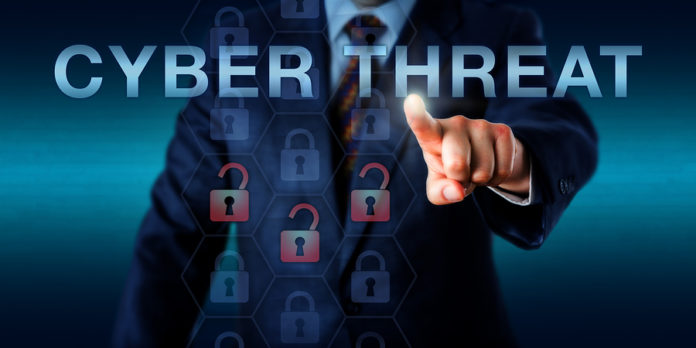
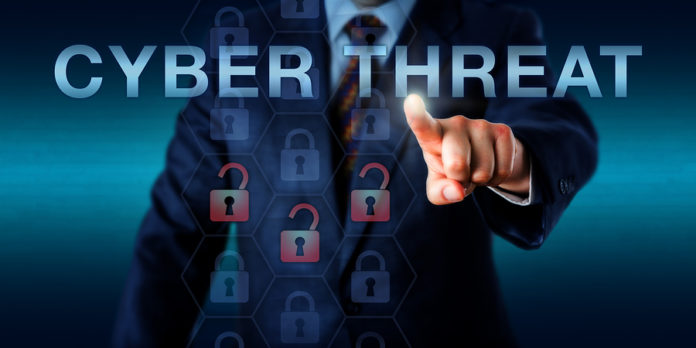
This post was originally published here by (ISC)² Management.
Whilst figures differ depending on which report you read, Gartner estimates the average time between a breach and detection to be about 285 days. By this time, an attacker has long gone. With all the security products in an enterprise network today, why is this still so long?
One reason maybe because threat detection is a big data problem. Particularly for network traffic based solutions. A handful of probes, or mirror ports, across a high-speed enterprise network and you could be capturing Terabytes of network packets a day. This then needs to be correlated to your SIEM. This all poses several problems. One, this data needs to be stored, potentially for a long time if you obliged to collect data for legal interception. But storage is relatively in-expensive in the larger scheme of things. Two, and more importantly, this data needs to be retrieved. How long does it take an analyst to sort through several months of PCAP to trace an intruder, even when the data is indexed. And three, SIEM data and packet capture data are unstructured, meaning they must be searched separately, or correlated before they can be cross-referenced.
The Cyber Security & Networking Research Group at Anglia Ruskin University are researching ways to tackle the big data challenges in threat detection. Our talk will present BotProbe. BotProbe started as a Ph.D/ project to improve the efficiency in capturing network traffic pertaining to botnet communications. Part of this addressed by the application of IPFIX. IPFIX is the ratified standard for flow export. IPFIX was designed to overcome the known drawbacks of network management based NetFlow for use in security processes such as threat detection. Through analysis of 20 million botnet flows we were able to create a template to capture the primary layer 3 to layer 7 fields in a packet pertaining to botnet communications. Our template realises a 97% reduction in traffic volumes over traditional packet capture. Furthermore, IPFIX is structured; meaning it can be indexed for easy retrieval, leading to a direct decrease in analysis time.
Our talk will focus on the BotProbe case study. However, our capture techniques don’t stop with botnet traffic. To date, we have applied adaptive data capture to malicious HTTP, SPAM, IoT device communication and Industrial Control Systems inter-device communications. Our talk will explain two direct benefits from such these levels of data reduction in threat detection. First, that it is now economical to capture and store network traffic data for pre-attack forensics. Secondly, with a reduced volume of data it becomes possible to apply machine-learning techniques to support SOC analysts in detection of suspicious behaviour within a network.
Photo:Billington CyberSecurity